YouTube Video Marketing Ideas
페이지 정보
작성자 Eric 작성일24-08-13 17:47 조회8회 댓글0건관련링크
본문
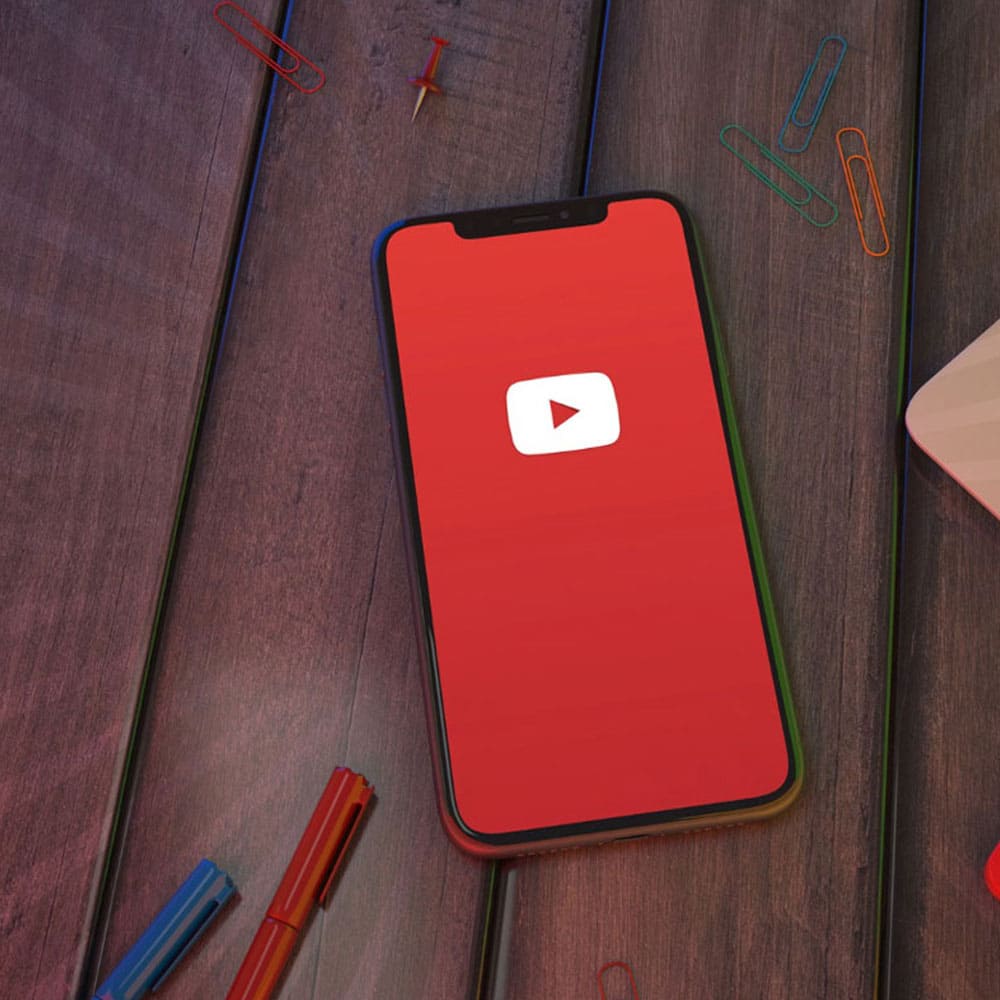
To appreciate the upcoming improvements, it's vital to comprehend the present state of YouTube's suggestion system. Historically, YouTube made use of fundamental metrics such as views, likes, and shares to suggest material. With time, the algorithm evolved to incorporate extra sophisticated signals, including watch time, individual interaction, and customized viewing practices. The intro of deep learning techniques additionally enhanced the algorithm's ability to predict user preferences and suggest appropriate content.
Today, the YouTube algorithm runs on a multi-layered structure. The first layer identifies a pool of prospective videos a user could such as, while the 2nd layer ranks these video clips based on numerous variables, consisting of customer history, interaction metrics, and contextual signals.
By evaluating user interactions as they happen, the formula can refine its forecasts and supply more exact material recommendations. An individual may like instructional content throughout weekdays and amusement on weekends. Adaptive discovering systems conquer this by using strategies such as federated knowing, which allows the formula to find out from decentralized data sources without jeopardizing customer privacy.
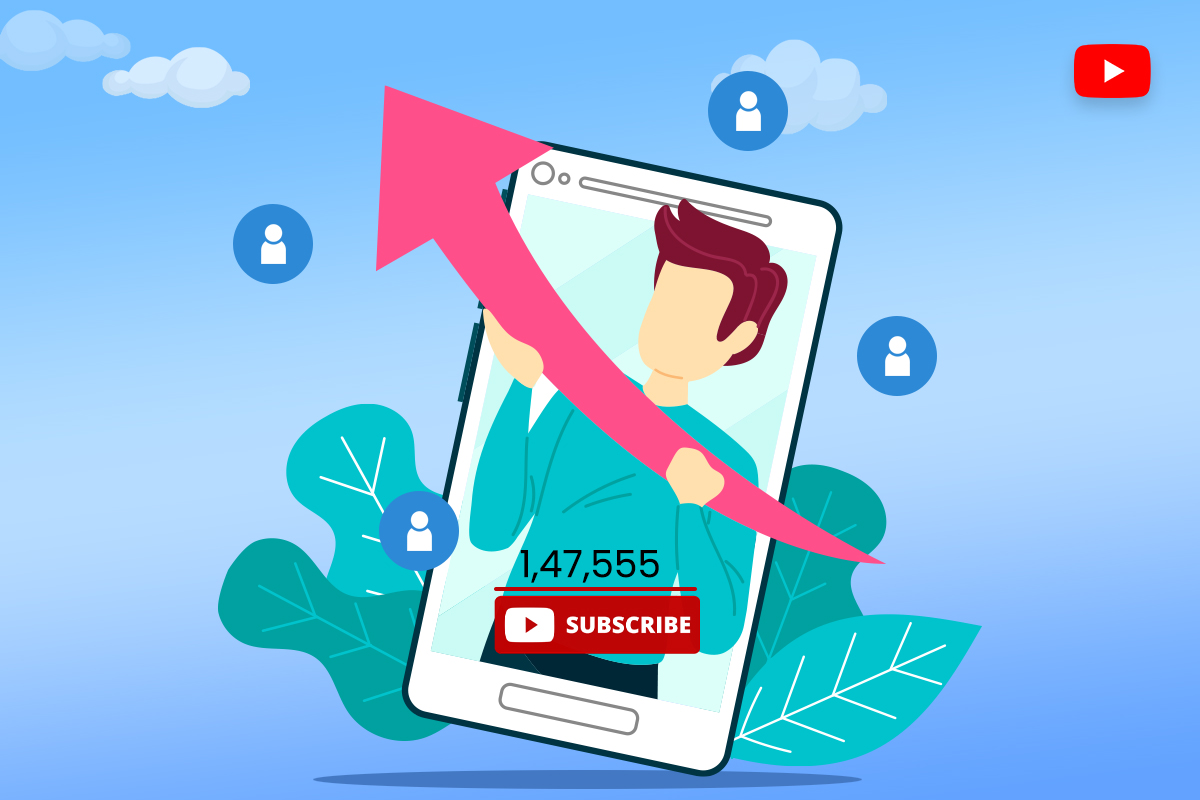
- Boosted Individual Engagement: By offering a lot more exact and contextually relevant suggestions, flexible knowing systems can significantly improve customer interaction. Users are more probable to locate content that resonates with their existing rate of interests and choices, leading to longer watch times and increased communication.
- Material Variety: One of the objections of existing referral systems is the creation of filter bubbles, where customers are continuously revealed similar web content, restricting their exposure to varied perspectives. Adaptive understanding systems can alleviate this by dynamically changing referrals to introduce a more comprehensive range of content, thus enhancing content diversity.
- Real-Time Adaptation: The capacity to adapt in real-time makes sure that the formula remains relevant also as customer preferences evolve. This is specifically vital in today's hectic digital landscape, where patterns and passions can alter rapidly. Real-time adaptation allows the algorithm to stay in advance of these shifts, keeping its efficiency over time.
- Improved Material Discovery: For material makers, adaptive knowing systems use a fairer chance at reaching their target market. By recognizing arising fads and user passions in real-time, the formula can advertise new and relevant content better. This enhances material exploration and gives developers with better chances to grow their target market.
Study: Execution and Outcomes
To illustrate the sensible influence of flexible knowing systems, take into consideration a theoretical case study where YouTube applies this technology.
Scenario: YouTube presents flexible discovering systems in a phased rollout, beginning with a subset of individuals in a particular area. The goal is to analyze the effect on individual involvement, web content variety, and overall contentment.
Technique: The rollout entails incorporating real-time comments loops, context-aware recommendations, and multi-modal information integration right into the existing formula. Individual interactions, watch times, and feedback are checked continually to evaluate the system's performance.
Outcomes:.
- User Engagement: Within the very first 3 months, customers in the test group reveal a 20% increase in average watch time and a 15% increase in interaction rates (likes, comments, shares) contrasted to the control team.
- Web content Diversity: Evaluation reveals a 25% boost in the diversity of content eaten by individuals in the examination team. Users are revealed to a more comprehensive variety of styles and designers, decreasing the incidence of filter bubbles.
- Customer Satisfaction: Surveys show a 30% enhancement in customer fulfillment scores, with users reporting that the suggestions feel extra relevant and personalized.
- Web content Exploration: New and arising content designers experience a 40% increase in exposure and involvement, as the formula a lot more efficiently promotes fresh and relevant content.
Obstacles and Future Directions
While the benefits of flexible learning systems are clear, their execution is not without obstacles. These include:.
- Computational Intricacy: Real-time adjustment calls for substantial computational resources, specifically for a platform as large as youtube marketing services (Storeboard official blog). Guaranteeing that the system can scale successfully is a vital obstacle.
- Information Privacy: Leveraging real-time user information increases personal privacy issues. Implementing durable privacy-preserving methods, such as differential personal privacy and federated discovering, is vital to attend to these worries.
- Predisposition and Justness: Ensuring that the algorithm continues to be unbiased and reasonable is a constant difficulty. Adaptive understanding systems need to be created to discover and reduce biases that can emerge from real-time data inputs.
Future directions for r & d include exploring a lot more advanced AI techniques, such as reinforcement understanding, to additionally enhance the flexibility and performance of recommendation systems. Furthermore, incorporating customer feedback more flawlessly right into the formula's learning process can provide beneficial understandings and boost its performance.
Verdict
The development of flexible knowing systems notes a significant leap in YouTube algorithm optimization, supplying an extra dynamic, context-aware, and personalized method to material referral. By dealing with the constraints of current algorithms and leveraging the most current innovations in AI and ML, these systems promise to boost user involvement, content diversity, and general satisfaction. As YouTube remains to advance, the integration of adaptive learning systems will most certainly play a crucial role fit the future of material discovery and referral.
These formulas are crucial to customer engagement, web content exploration, and system growth. The intro of deep learning strategies better enhanced the formula's capacity to predict user choices and advise pertinent content.
The initial layer recognizes a swimming pool of possible videos an individual could such as, while the 2nd layer places these videos based on countless aspects, including individual background, interaction metrics, and contextual signals. By acknowledging arising trends and individual passions in real-time, the formula can promote new and pertinent web content extra efficiently. By dealing with the limitations of current algorithms and leveraging the newest developments in AI and ML, these systems guarantee to improve individual involvement, web content diversity, and overall contentment.
댓글목록
등록된 댓글이 없습니다.